A machine learning algorithm can use X-ray diffraction data from polymers to predict the behaviour of new materials.
Published : August 15 ,2024 / DOI : 10.1080/14686996.2024.2388016
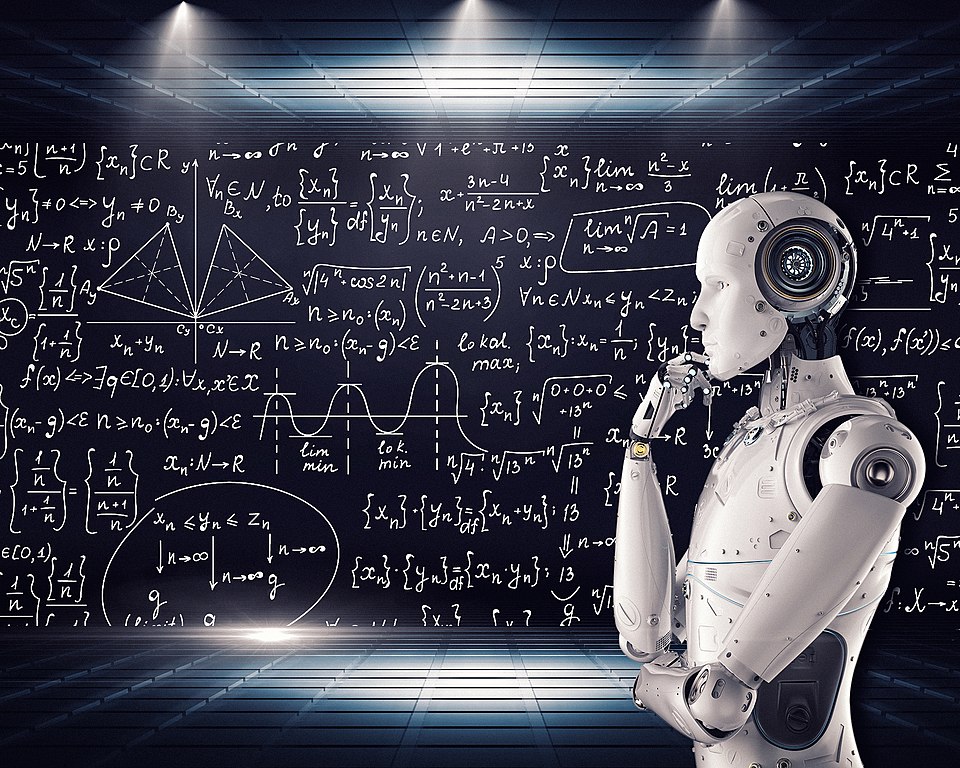
Polymers such as polypropylene are fundamental materials in the modern world, found in everything from computers to cars. Because of their ubiquity, it’s vital that materials scientists know exactly how each newly developed polymer will perform under different preparation conditions. Thanks to a new study, which was published in Science and Technology of Advanced Materials, scientists can now use machine learning to determine what to expect from a new polymer.
Predicting the mechanical properties of new polymers, such as their tensile strength or flexibility, usually involves putting them through destructive and costly physical tests. However, a team of researchers from Japan, led by Dr. Ryo Tamura, Dr. Kenji Nagata, and Dr. Takashi Nakanishi from the National Institute for Materials Science in Tsukuba, showed that machine learning can predict the material properties of polymers. They developed the method on a group of polymers called homo-polypropylenes, using X-ray diffraction patterns of the polymers under different preparation conditions to provide detailed information about their complex structure and features.
“Machine learning can be applied to data from existing materials to predict the properties of unknown materials,” Drs. Tamura, Nagata, and Nakanishi explain. “However, to achieve accurate predictions, it’s essential to use descriptors that correctly represent the features of these materials.”
Thermoplastic crystalline polymers, such as polypropylene, have a particularly complex structure that is further altered during the process of molding them into the shape of the end product. It was, therefore, important for the team to adequately capture the details of the polymers’ structure with X-ray diffraction and to ensure that the machine learning algorithm could identify the most important descriptors in that data.
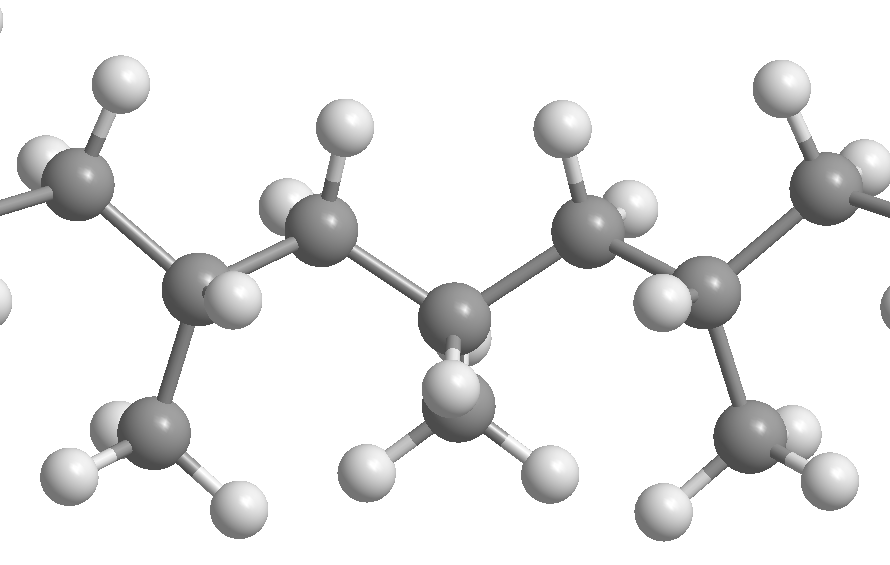
To that end, they analysed two datasets using a tool called Bayesian spectral deconvolution, which can extract patterns from complex data. The first dataset was X-ray diffraction data from 15 types of homo-polypropylenes subjected to a range of temperatures, and the second was data from four types of homo-polypropylenes that underwent injection molding. The mechanical properties analysed included stiffness, elasticity, the temperature at which the material starts to deform, and how much it would stretch before breaking.
The team found that the machine learning analysis accurately linked features in the X-ray diffraction imagery with specific material properties of the polymers. Some of the mechanical properties were easier to predict from the X-ray diffraction data, while others, such as the stretching break point, were more challenging.
“We believe our study, which describes the procedure used to provide a highly accurate machine learning prediction model using only the X-ray diffraction results of polymer materials, will offer a nondestructive alternative to conventional polymer testing methods,” the NIMS researchers say.
The team also suggested that their Bayesian spectral deconvolution approach could be applied to other data, such as X-ray photoelectron spectroscopy, and used to understand the properties of other materials, both inorganic and organic.
“It could become a test case for future data-driven approaches to polymer design and science,” the NIMS team says.
Further information:
Ryo Tamura
National Institute for Materials Science (NIMS)
tamura.ryo[at]nims.go.jp
Kenji Nagata
National Institute for Materials Science (NIMS)
nagata.kenji[at]nims.go.jp
Takashi Nakanishi
National Institute for Materials Science (NIMS)
nakanishi.takashi[at]nims.go.jp
Paper: https://doi.org/10.1080/14686996.2024.2388016
About Science and Technology of Advanced Materials (STAM)
Open access journal STAM publishes outstanding research articles across all aspects of materials science, including functional and structural materials, theoretical analyses, and properties of materials. https://www.tandfonline.com/STAM
Dr Yasufumi Nakamichi
STAM Publishing Director
Email: NAKAMICHI.Yasufumi[at]nims.go.jp
Information
- Authors
- Ryo Tamura, Kenji Nagata, Keitaro Sodeyama, Kensaku Nakamura, Toshiki Tokuhira, Satoshi Shibata, Kazuki Hammura, Hiroki Sugisawa, Masaya Kawamura, Teruki Tsurimoto, Masanobu Naito, Masahiko Demura & Takashi Nakanishi
- Citation
- Sci. Technol. Adv. Mater. Vol. 25 (2024) 2388016
- URL
- https://doi.org/10.1080/14686996.2024.2388016